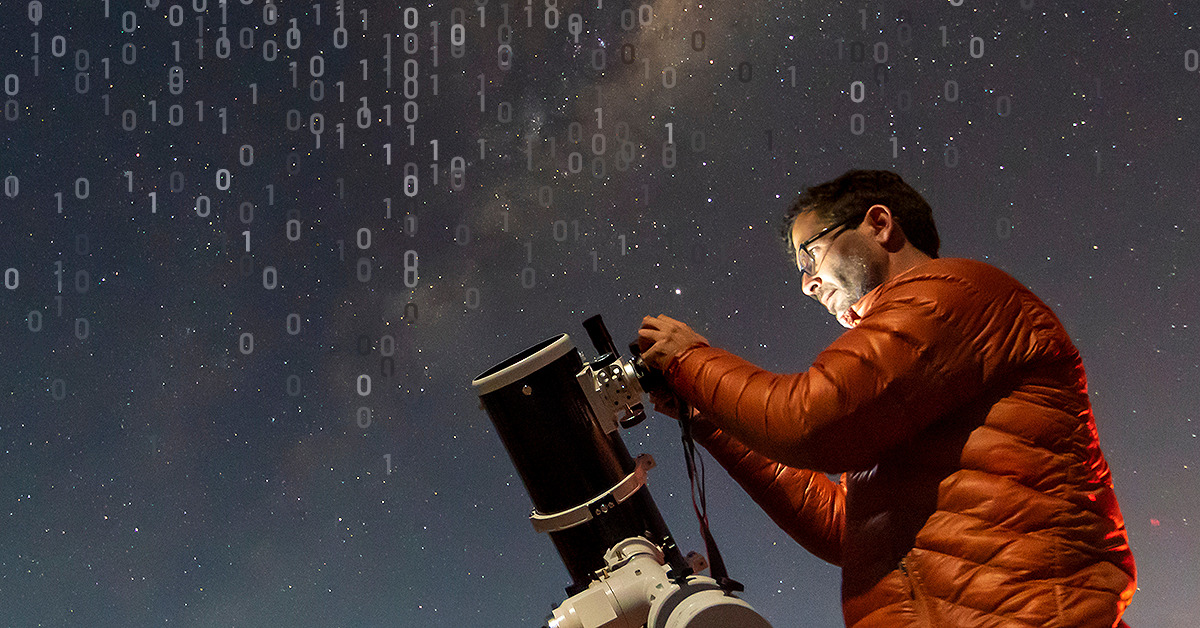
Data management trends to watch in coming years
Everybody talks about data and data-driven enterprise. Without a doubt, data-related topics will dominate technology trends for the coming years. That is why we decided to take a look at 5 hot topics that we think you should be keeping an eye on.
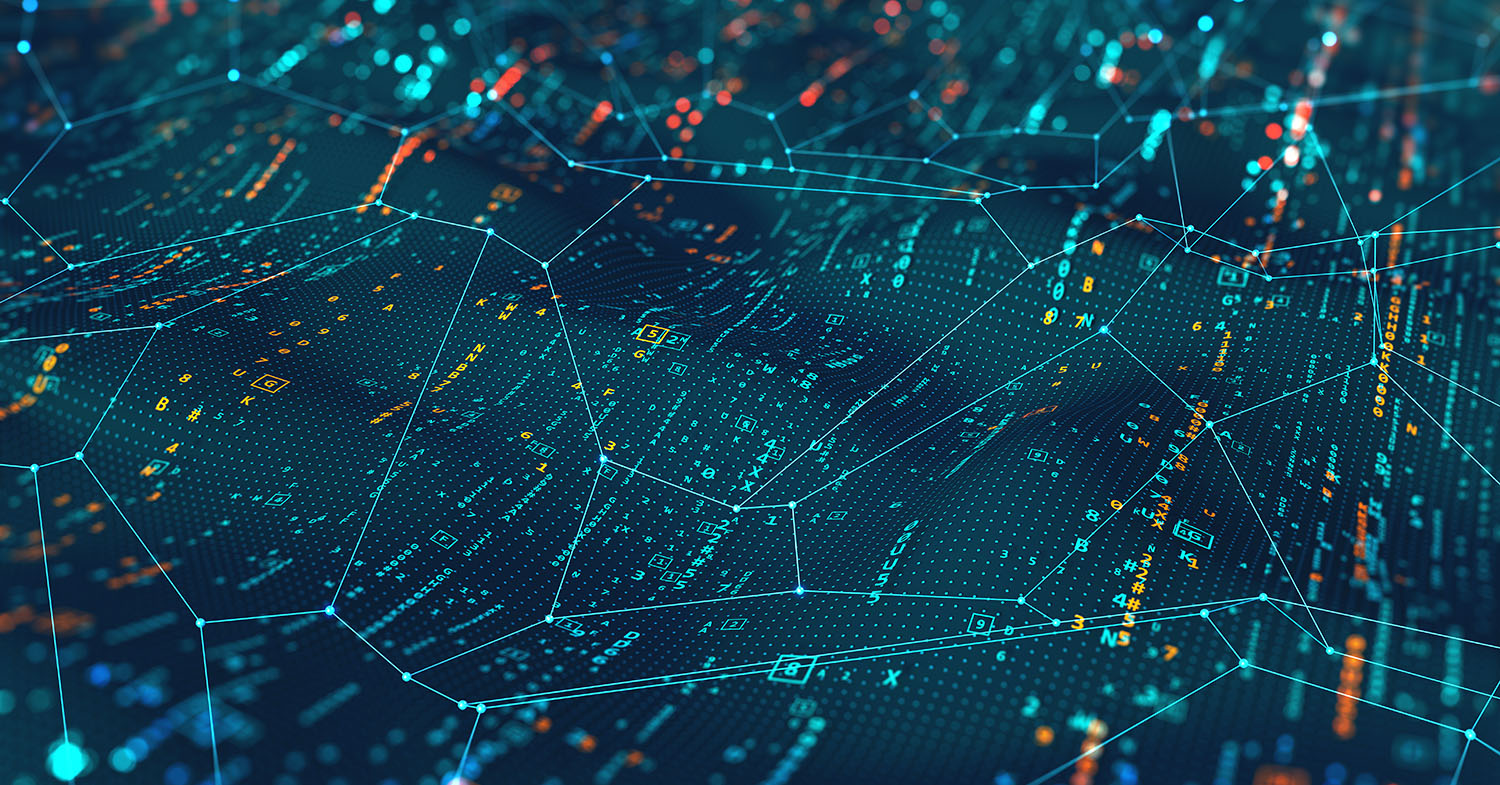
Data Fabric
Data Fabric is an emerging concept for managing massive amounts of data distributed among numerous disconnected sources in on-premises, multi-cloud and hybrid environments.
One of the primary goals of data fabric is to maximize the business value of information by data democratization. With data fabric, data consumers across the organization, including business analysts, gain easy access to reliable data consolidated from all sources exactly when they need it.
As a result, they no longer have to investigate where the information they require is stored, who owns it, what its quality is or in what format it is available. They can quickly conduct some analysis, validate a business idea or start some other activity without waiting a long time for relevant data to be collected, consolidated and properly prepared.
Data fabric is not a technology or a single product. It is a combination of a certain concept of data architecture and a set of tools necessary to implement that concept. Moreover, there is no single universal architecture pattern for data fabric. Different software vendors present their own perspective on this concept, tailored primarily to their solutions.
An important feature of this architecture is modularity, which makes it extremely flexible. When composing a data platform to support data fabric, an organization can incorporate any capabilities, including data governance and privacy, compliance, data security, data analytics, data integration, data quality, data transformation, data enrichment, data preparation, or whatever is needed.
Another major advantage of this approach is the ability to leverage both the existing data infrastructure and new tools and technologies. Data fabric thus allows for the evolutionary modernization of an organization’s data environment by gradually replacing legacy software with newer solutions.
The basic assumption of data fabric is to leave the data in its original source, where its consistency and high quality are ensured.
This is made possible through data virtualization. Instead of creating another central repository and replicating or moving data to it, a data catalog is used that scans all sources for metadata. This creates a virtual index of all data assets from all sources available in the organization, regardless of location, type, format or technology.
With such a catalog, users get full insight into what data assets a company has, for example, what range of customer data is available across different sources. Users can search, filter and sort data according to multiple criteria, including data quality metrics. In this way, they can, for instance, see which source offers the most up-to-date and reliable set of customer emails.
Every single action is performed, naturally, in accordance with data governance, privacy and security.
Data is accessed through the data marketplace. Here, users can not only browse available data assets, but also order a specific dataset, for example, for a marketing or sales campaign.
The name not coincidentally refers to an online store. Data marketplaces work quite similarly. A data consumer browses the available resources, adds the necessary data sets to the basket, and then places an order to have the merged data package delivered to him in the required format, such as an Excel spreadsheet.
Data Fabric is often referred to as a solution for breaking down data silos. But what does this mean in practice?
“Data fabric does not modify or replace the original data repositories.” – explains Krzysztof Wiśniewski, Senior System Architect at Striped Giraffe. “It can be seen as a sort of fabric overlaid on top of all existing data sources, interconnecting them and enabling their functioning as a single virtual environment. Thus, from the data consumer’s perspective, data silos disappear. There is only one central, metadata-driven catalog of all data which can be browsed and queried through the data marketplace. You can say that data fabric is the cure for all the headaches caused by siloed data without physically eliminating the silos themselves.”
Within the services that make up the data fabric architecture, most processes and dataflows can be automated. This significantly increases efficiency and, in many cases, allows users, systems and apps to access the required data in real time.
Gartner estimates that data fabric can reduce by 70% various data management tasks, including design, deployment and operations.
Many data experts indicate that data fabric is the future of data management. According to Gartner, by 2024, data fabric deployments will quadruple the efficiency of data usage while cutting human data management tasks in half.
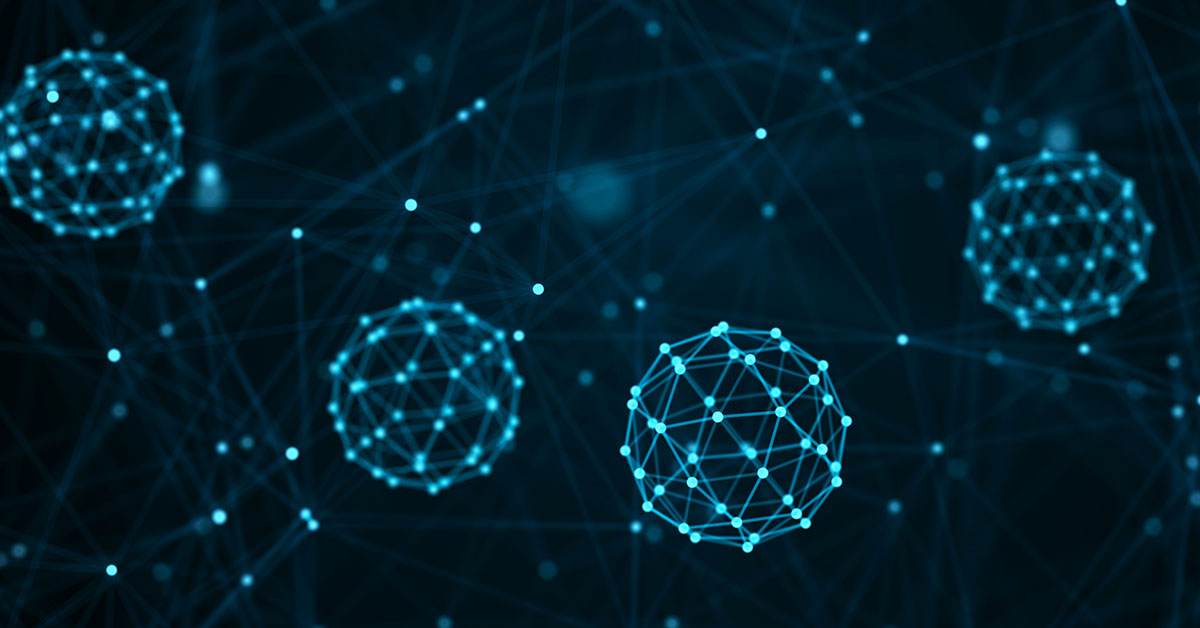
Data Mesh
Data mesh is another emerging concept for building a data architecture in an environment with heterogeneous data sources.
At first glance, data fabric and data mesh look quite similar. The fundamental difference is that data mesh represents a decentralized and domain-oriented approach to data architecture. In contrast, data fabric manages multiple data sources as if it were a single virtual and centralized data system in which data domains are of little relevance.
For example, instead of a single domain bringing together marketing, sales, financial, human resources and logistics data, in a data mesh architecture each of these data sets is separated into a different domain.
Data mesh relies on the following four key principles:
Domain ownership
Unlike data fabric, there is no single central team responsible for governing and managing all data and making it available to data consumers in the data marketplace. Ownership of analytics and operational data is transferred to domain teams.
Domain teams are responsible for the entire lifecycle of data products within their domain. For example, one team is responsible only for the financial data domain, another for Human Resources data, and yet another for CRM data.
The domain team itself performs the analytics needed to make data-driven decisions. If necessary, it can obtain the information it requires from other domains.
Data as a product
In a data mesh, data is treated as a product that has its consumers. The assumption is that outside of a given domain, somewhere in the organizational structure, there are teams (which may be other domain teams) that are consumers of this data and need it for analytics.
Thus, the primary responsibility of the domain team is to create appropriate data products to meet the needs of data consumers outside the team. The domain team must ensure that its data is of high quality and accessible to others.
A data product is usually a domain-specific dataset described by metadata, which facilitates its discovery, access and use. This metadata includes ownership and contact information, data location and access, update frequency, and data model specification.
In the data marketplace, data consumers can browse data products directly within the domain of their interest and order the necessary data to be prepared for them by the relevant domain team.
Self-service data platform
As with data fabric, an inherent part of the data mesh concept is a self-service data platform. It provides all the necessary capabilities, including storage, data retrieval, processing, analysis, visualization, metadata and permissions management, etc.
The data platform provides domain teams with the necessary tools to:
- ensure the quality of their data
- easily build their own data products and make them available to data consumers
- perform data evaluation
- create data visualizations
- acquire data from other domains
- and effectively conduct their own analysis.
Federated governance
Although data governance and management across individual domains are distributed among the domain teams, there is an overarching federated governance team that plays a supervisory and coordinating role.
It typically comprises representatives from all the teams involved, including IT. Together, they agree on standards and common policies for interoperability, documentation, security, privacy and compliance. These policies form the rules for operating in the data mesh, in particular, how domain teams should build their data products.
In many use cases, the federated governance team is also responsible for all those data elements that are shared across domains. Often, when some data is duplicated in several domains, it is moved under the federated governance team’s management. In this way, duplications are removed from the domain-specific datasets.
Data mesh focuses primarily on use cases of data for analytical, not operational, purposes. Therefore, it does not address data products used to serve real-time needs.
Data mesh is a natural choice for organizations where the IT systems environment is modularized according to the domain-driven design principles or similar. This does not mean that it cannot be adopted by companies operating on data silos, such as data warehouses or data lakes. It is still possible, provided that the relevant areas of data domains are separated and managed by dedicated teams within these silos.
The prerequisite for data mesh is to have a sufficient number of independent domain teams that already have their systems running in production. However, if your organization is too small and you do not have many independent teams, you should rather avoid this approach.
Like data fabric, data mesh is not a ready-made product or technology. There is no one-size-fits-all model of the architecture. There are only general assumptions and guidelines that define the whole concept. Therefore, each organization can design it to best fit its specifics and needs.
Importantly, both approaches – data fabric and data mesh – can be implemented using the same tools. The difference lies in modeling the entire architecture, organizing the teams in a different way and implementing different processes within the company.
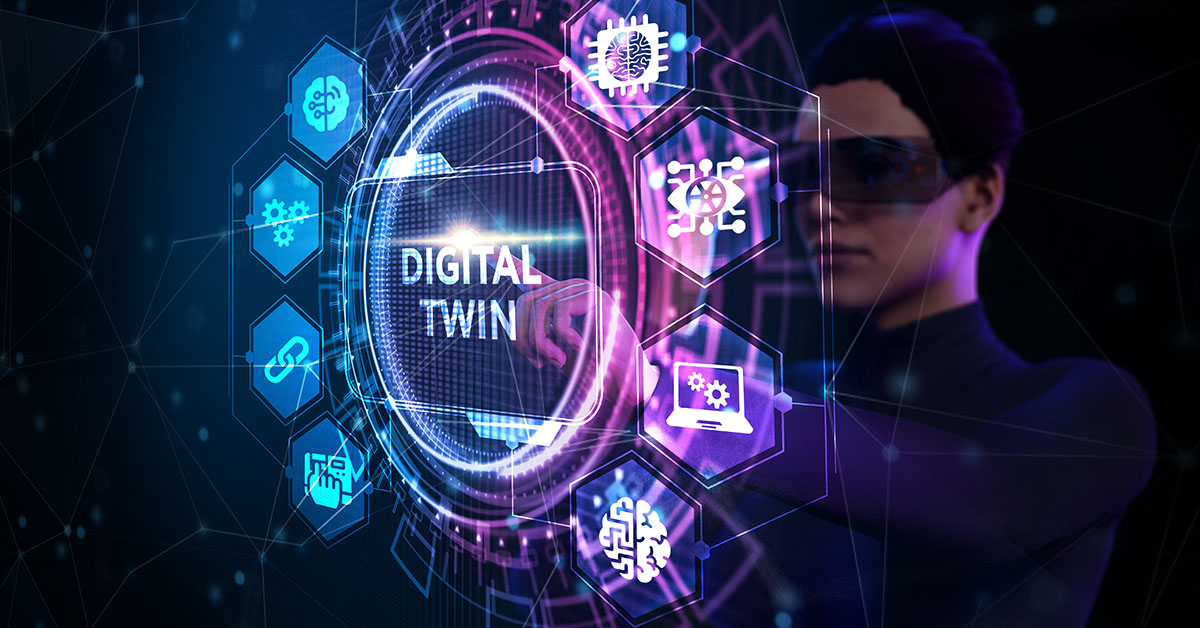
Digital twin of the customer
The concept of a digital twin is well known and successfully applied for years in industrial manufacturing. It leverages the Internet of Things (IoT) and machine learning to create virtual replicas of real objects – machines, devices, production lines, etc. It is also increasingly used to monitor and model processes.
In recent years, this concept has found application in many other industries, such as construction, logistics, automotive, medicine, healthcare, aviation, public administration and even veterinary medicine. Currently, it is gaining momentum in commerce, offering the promise of delivering a digital model of the customer with their preferences, emotions and shopping behavior.
At first glance, this concept resembles Customer 360 View, which can be established with a Customer Data Platform. Indeed, the starting point for creating a digital twin is to collect comprehensive customer data from all available sources. However, the digital twin goes much further and incorporates artificial intelligence and machine learning to simulate customer behavior and adjust these simulations in real time.
The key to creating such a self-learning model is behavioral data from customer journey analysis. For this purpose, all customer interactions with a brand are monitored, both in digital and offline channels. Other data may also be used, such as geolocation.
Obviously, you cannot attach sensors to a human, nor track a person 24 hours a day. Therefore, it is essential to create as many customer-brand touch points as possible across all channels. In doing so, you must have the ability to digitally capture all interactions and extract useful data from them.
In order to get as much data as possible, you also need to have an idea of how to encourage the customer to interact at these points and thus share information with you.
In addition, everything must be done with the full awareness and consent of the customer. For this, a proper privacy policy and consent management are required.
To ensure the accuracy of the model, it is not enough to collect a sufficiently large volume of data. Its quality is equally important. Duplicate, incomplete, incorrect or outdated data can distort the customer’s picture and create a false model that is useless or even harmful to your business. Therefore, a comprehensive data quality management system (DQMS) is a must-have if you are thinking about a digital twin.
For the required data quality, you must be able to precisely determine the customer identity. This involves being able to correctly attribute recorded interactions to specific individuals. This can be a major challenge as the customer may use different authentication methods for different interactions with your brand, e.g., different emails, phone numbers or even postal addresses. To handle this issue, you need strong identity resolution capabilities.
“Customer tastes and behaviors change quickly and frequently, so the digital twin of the customer must evolve with them.” – explains Krzysztof Wiśniewski. “This requires a constant supply of new data and adjusting the machine learning model accordingly. It is a closed cycle. New data improves and calibrates the model, while this evolving model is used to shape future interactions that provide new data.”
Among the many benefits of implementing the digital twin of the customer, the most important are:
- better understanding of customer motivation – why they engage and buy from you
- emulating and predicting customer behavior
- modifying and enriching customer experience
- improving personalization
- more accurate recommendations
- designing optimal purchase journeys
- testing marketing campaigns
- choosing the best shopping incentives
- testing new products and services.
The digital twin of the customer holds a great deal of potential. It will evolve over time with advances in artificial intelligence and machine learning technologies.
What direction is this concept heading? Gartner’s Digital Disruption Scale brings the answer.
As for 2022, on a five-point scale, Gartner placed the digital twin of the customer almost at the beginning of its journey, somewhere between enhancing (level 1) and extending markets (level 2). It will take five more years to reach level 4, when it will become the driving force behind reinventing markets.
Gartner also predicts that by 2030 the market for software and services that enable digital twins will reach $150 billion globally, up from $9 billion in 2022.
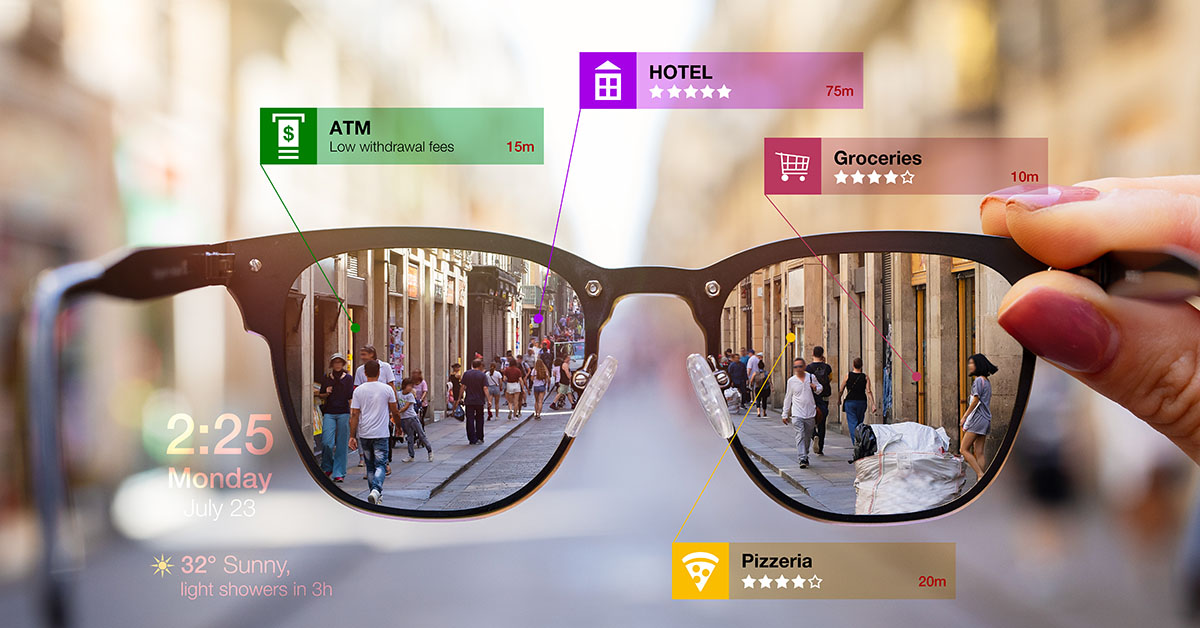
AR and VR data
Thanks to the rapid development of digital reality technologies, we are able to digitally enhance almost every sphere of our private or professional lives with data or metadata. In this way, companies can use the wealth of information they have to offer customers a whole new dimension of experience. But this is not the only use and benefit of this technology.
Currently, there are three leading types of digital realities:
- Augmented reality (AR) – involves superimposing digitally generated 3D images, sound elements or other sensory information on the real world. A mobile device is usually required to use it, so the ability to interact with the digital dimension is (at least for now) limited to the screen of a smartphone or tablet.
- Virtual reality (VR) – creates a completely digital and fully interactive 3D environment in which the user is immersed and isolated from the real world. VR headsets containing goggles with built-in headphones and microphone are most often required. In addition to creating fictional worlds, VR can also be used to develop digital copies of real locations. This allows users, for example, to visit a museum, walk through a store, warehouse or production facility as if they were actually there.
- Mixed Reality (MR) – uses holographic lenses (e.g., in smart glasses) to combine VR and AR elements with the real world so that digital objects can interact with real objects.
Since AR does not require specialized equipment, it has quickly become the most popular and common of the three mentioned above. It is safe to say that it can be used by literally everyone.
The popularization of this technology is also fostered by the increasing choice and affordability of software tools to implement it. As a result, companies do not need to invest huge amounts of money in their own solutions to quickly make use of the data they have.
AR is most widely used in retail, where it enhances the customer experience both in and out of stores. For example, it allows shoppers to view products in their homes before making a purchase – to virtually try on clothes, see if new furniture will fit in a room, or choose the right color for painting walls.
This technology also finds application in many other industries and spheres of life, such as marketing, manufacturing, healthcare, automotive, product development, tourism and education.
Using existing data to enhance reality is one thing. Another is the wide spectrum of data that can be extracted from human interaction with both augmented and virtual reality. The way a user interacts with digital world makes it possible to obtain a lot of invaluable information about their preferences (e.g., when choosing products), behavior patterns (e.g., when visiting a virtual store), skills (e.g., during virtual training), the way they use products or devices, etc.
This data, properly processed and used, allows companies to transform how they make sales, serve their customers, arrange space in stores, create products and services, design processes, train employees, etc.
It is no exaggeration to say that digital realities still have their best days ahead of them. The breakthrough could be the improvement and popularization of holographic technology, such as the ability to switch ordinary glasses into AR/VR goggles. Or the ability to display holographic images in space and freely interact with them, which is known from SF movies, but not at all unrealistic today.
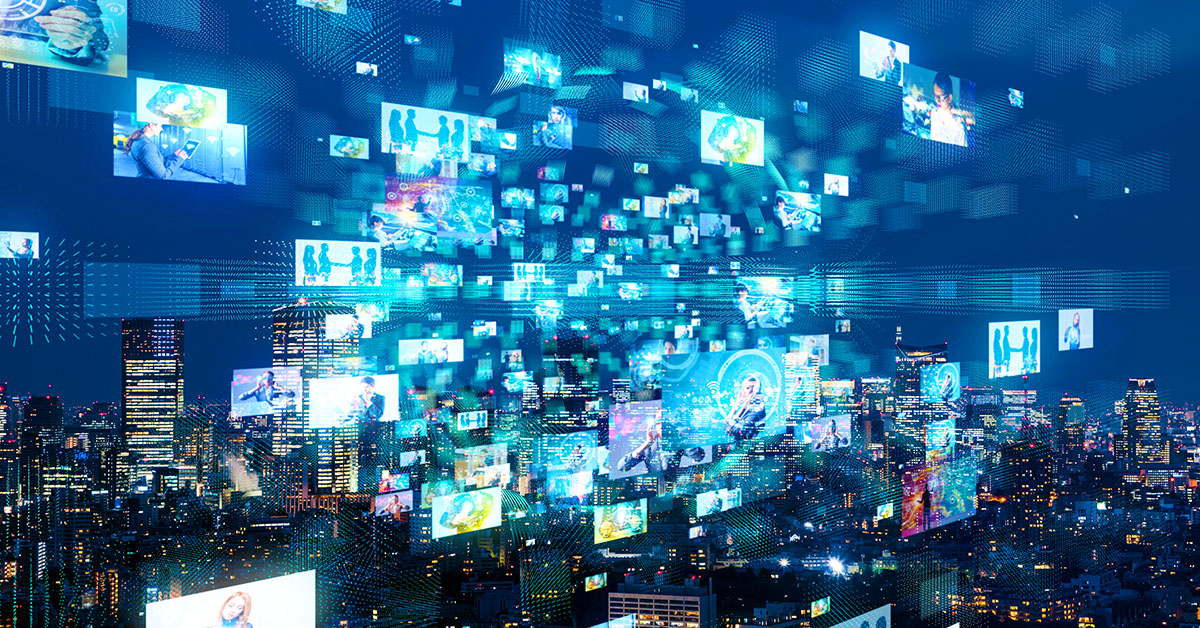
Increasing need for handling unstructured data
Most of the information generated in today’s world is unstructured data. Various studies indicate that they can account for as much as 80-90% of all data. In addition, unstructured data is the fastest-growing category of data.
The most common type of unstructured data is text, but it also comes in the form of images and audio and video files. Examples of such data include:
- full texts of emails
- customer reviews, comments and opinions
- posts, images and videos from blogs and social media
- transcripts from meetings
- conversations from discussion forums
- online chats
- customer service calls
- logs from websites, servers, networks and applications
- data from sensors on IoT-connected devices.
Unlike structured data, unstructured data does not have a predefined data model or schema. Therefore, they cannot be easily stored in a traditional relational database, table or spreadsheet based on a column-row structure of information. They are also not easily classifiable, for instance, it is not possible to clearly determine whether some piece of data is of integer, date, time or other type.
All of this makes unstructured data the most challenging type of data to handle – from collecting and storing, to processing, searching and analyzing.
Not so long ago, unstructured data was almost useless to organizations. The main reason was the lack of appropriate technologies and software to effectively process and analyze such information.
Today, however, companies have access to numerous tools that support natural language processing, speech-to-text conversion, image recognition, sentiment analysis, pattern recognition, and other technologies powered by artificial intelligence and machine learning. They allow them to identify and extract information from huge sets of unstructured data, analyze it and derive valuable insights.
In practice, unstructured data can contain a wealth of invaluable information, including structured data mixed with other content. For example, a transcript of a phone call with a customer may feature their personal details, expectations regarding price and terms of sale, or even information about their purchases from your competitors.
In many cases, unstructured data is the missing piece of the puzzle to put the whole together and gain full insight into specific topics.
“For example, without unstructured data, it is difficult for companies to get a complete view of a customer.” – Krzysztof Wiśniewski says. “You may know all the personal details of a user, their purchase history, products and content browsed, but this will not let you derive reliable insights about the customer’s attitude towards your brand. The information you need is encoded in customer-generated content mainly on social media and discussion forums.”
Simply put, without unstructured data analyzed with AI/ML, you have no chance of creating a truly complete view of the customer (Customer 360), much less a digital twin of the customer.
The same goes for your products and services. Collecting and analyzing what customers say about your offerings – their comments, reviews, photos or videos – will allow you to better understand the strengths and weaknesses of your proposition and, as a result, make more accurate decisions about future developments.
The tremendous business value of unstructured data can be uncovered across all industries and countless use cases, including:
- fraud detection in the financial and insurance industries
- disease diagnosis and determining treatment methods in healthcare
- identifying trends, such as customer purchasing trends
- early detection of malfunctions in equipment, e.g., engines, production machines, power tools
- determining a customer’s propensity to purchase a specific product
- improving call center services through semantic analytics of customer conversations.
The potential of unstructured data lies not only in the vast amount of information that can be extracted, but also in the fact that still few organizations have entered the field.
A survey by Deloitte revealed that most organizations (64%) relied on structured data from internal sources, while only 18% used unstructured data. Another study showed that currently only 0.5% of unstructured data is analyzed.
What does this mean for your organization? That by getting down to the subject right now, you can quickly gain a significant advantage over your competitors.
This might also be of interest to you:
BLOG
Approaching customers digitally with the Customer Data Platform (CDP)
Customer centricity is essential when it comes to establishing digital business models. It requires the best possible knowledge of where those customers currently are in their individual customer journey.
E-BOOK
Cloud DWH: Next-Generation Data Management
Companies today are increasingly data-driven as part of the digital transformation. This enables them to an acceleration of time-to-market, a reduction in costs, a significant increase in efficiency as well as the generation of reliable insights for business decision-making.
BLOG
Data governance – the linchpin of efficient data management
Companies that want to set up their data management properly in order to make efficient use of one of their most valuable assets – their data – need professional data governance. You can’t do it without it.
CASE STUDY
Ratioform: Next Level Product Management With Machine Learning
Obtaining meaningful information about products was a complex task, which distracted the focus from the value-adding activities of a product manager. The Machine Learning based solution implemented was able to overcome this challenge.